Python機器學習三大件之二pandas
2008年WesMcKinney開發出的庫
專門用于數據挖掘的開源python庫
以Numpy為基礎,借力Numpy模塊在計算方面性能高的優勢
基于matplotlib,能夠簡便的畫圖
獨特的數據結構
二、數據結構 Pandas中一共有三種數據結構,分別為:Series、DataFrame和MultiIndex。三、SeriesSeries是一個類似于一維數組的數據結構,它能夠保存任何類型的數據,比如整數、字符串、浮點數等,主要由一組數據和與之相關的索引兩部分構成。
import pandas as pdpd.Series(np.arange(3))
0 01 12 2dtype: int64
#指定索引pd.Series([6.7,5.6,3,10,2], index=[1,2,3,4,5])
1 6.72 5.63 3.04 10.05 2.0dtype: float64
#通過字典數據創建color_count = pd.Series({’red’:100, ’blue’:200, ’green’: 500, ’yellow’:1000})color_count
blue 200green 500red 100yellow 1000dtype: int64
Series的屬性color_count.indexcolor_count.values
也可以使用索引來獲取數據:
color_count[2]
100
Series排序data[‘p_change’].sort_values(ascending=True) # 對值進行排序data[‘p_change’].sort_index() # 對索引進行排序#series排序時,只有一列,不需要參數
四、DataFrame創建
pd.DataFrame(np.random.randn(2,3))
score = np.random.randint(40, 100, (10, 5))score
array([[92, 55, 78, 50, 50],[71, 76, 50, 48, 96],[45, 84, 78, 51, 68],[81, 91, 56, 54, 76],[86, 66, 77, 67, 95],[46, 86, 56, 61, 99],[46, 95, 44, 46, 56],[80, 50, 45, 65, 57],[41, 93, 90, 41, 97],[65, 83, 57, 57, 40]])
但是這樣的數據形式很難看到存儲的是什么的樣的數據,可讀性比較差!!
# 使用Pandas中的數據結構score_df = pd.DataFrame(score)
data.shapedata.indexdata.columnsdata.valuesdata.Tdata.head(5)data.tail(5)data.reset_index(keys, drop=True)keys : 列索引名成或者列索引名稱的列表drop : boolean, default True.當做新的索引,刪除原來的列
dataframe基本數據操作data[‘open’][‘2018-02-27’] # 直接使用行列索引名字的方式(先列后行)data.loc[‘2018-02-27’:‘2018-02-22’, ‘open’] # 使用loc:只能指定行列索引的名字data.iloc[:3, :5 ]# 使用iloc可以通過索引的下標去獲取data.sort_values(by=“open”, ascending=True) #單個排序data.sort_values(by=[‘open’, ‘high’]) # 按照多個鍵進行排序data.sort_index() # 對索引進行排序
DataFrame運算
應用add等實現數據間的加、減法運算應用邏輯運算符號實現數據的邏輯篩選應用isin, query實現數據的篩選使用describe完成綜合統計使用max, min, mean, std完成統計計算使用idxmin、idxmax完成最大值最小值的索引使用cumsum等實現累計分析應用apply函數實現數據的自定義處理
五、pandas.DataFrame.plotDataFrame.plot(kind=‘line’)kind : str,需要繪制圖形的種類‘line’ : line plot (default)‘bar’ : vertical bar plot‘barh’ : horizontal bar plot關于“barh”的解釋:http://pandas.pydata.org/pandas-docs/stable/reference/api/pandas.DataFrame.plot.barh.html‘hist’ : histogram‘pie’ : pie plot‘scatter’ : scatter plot
六、缺失值處理isnull、notnull判斷是否存在缺失值np.any(pd.isnull(movie)) # 里面如果有一個缺失值,就返回Truenp.all(pd.notnull(movie)) # 里面如果有一個缺失值,就返回Falsedropna刪除np.nan標記的缺失值movie.dropna()fillna填充缺失值movie[i].fillna(value=movie[i].mean(), inplace=True)replace替換wis.replace(to_replace='?', value=np.NaN)
七、數據離散化p_change= data[’p_change’]# 自行分組,每組個數差不多qcut = pd.qcut(p_change, 10)# 計算分到每個組數據個數qcut.value_counts()
# 自己指定分組區間bins = [-100, -7, -5, -3, 0, 3, 5, 7, 100]p_counts = pd.cut(p_change, bins)
得出one-hot編碼矩陣
dummies = pd.get_dummies(p_counts, prefix='rise')#prefix:分組名字前綴八、數據合并
pd.concat([data1, data2], axis=1)按照行或列進行合并,axis=0為列索引,axis=1為行索引
pd.merge(left, right, how=‘inner’, on=None)
可以指定按照兩組數據的共同鍵值對合并或者左右各自left: DataFrameright: 另一個DataFrameon: 指定的共同鍵how:按照什么方式連接
九、交叉表與透視表交叉表:計算一列數據對于另外一列數據的分組個數 透視表:指定某一列對另一列的關系
#通過交叉表找尋兩列數據的關系count = pd.crosstab(data[’week’], data[’posi_neg’])#通過透視表,將整個過程變成更簡單一些data.pivot_table([’posi_neg’], index=’week’)十、數據聚合
count = starbucks.groupby([’Country’]).count()col.groupby([’color’])[’price1’].mean()#拋開聚合談分組,無意義
到此這篇關于Python機器學習三大件之二pandas的文章就介紹到這了,更多相關Python pandas內容請搜索好吧啦網以前的文章或繼續瀏覽下面的相關文章希望大家以后多多支持好吧啦網!
相關文章:
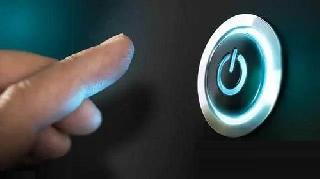