Python 實現 3 種回歸模型(Linear Regression,Lasso,Ridge)的示例
公共的抽象基類
import numpy as npfrom abc import ABCMeta, abstractmethodclass LinearModel(metaclass=ABCMeta): ''' Abstract base class of Linear Model. ''' def __init__(self): # Before fit or predict, please transform samples’ mean to 0, var to 1. self.scaler = StandardScaler() @abstractmethod def fit(self, X, y): '''fit func''' def predict(self, X): # before predict, you must run fit func. if not hasattr(self, ’coef_’): raise Exception(’Please run `fit` before predict’) X = self.scaler.transform(X) X = np.c_[np.ones(X.shape[0]), X] # `x @ y` == `np.dot(x, y)` return X @ self.coef_
Linear Regression
class LinearRegression(LinearModel): ''' Linear Regression. ''' def __init__(self): super().__init__() def fit(self, X, y): ''' :param X_: shape = (n_samples + 1, n_features) :param y: shape = (n_samples]) :return: self ''' self.scaler.fit(X) X = self.scaler.transform(X) X = np.c_[np.ones(X.shape[0]), X] self.coef_ = np.linalg.inv(X.T @ X) @ X.T @ y return self
Lasso
class Lasso(LinearModel): ''' Lasso Regression, training by Coordinate Descent. cost = ||X @ coef_||^2 + alpha * ||coef_||_1 ''' def __init__(self, alpha=1.0, n_iter=1000, e=0.1): self.alpha = alpha self.n_iter = n_iter self.e = e super().__init__() def fit(self, X, y): self.scaler.fit(X) X = self.scaler.transform(X) X = np.c_[np.ones(X.shape[0]), X] self.coef_ = np.zeros(X.shape[1]) for _ in range(self.n_iter): z = np.sum(X * X, axis=0) tmp = np.zeros(X.shape[1]) for k in range(X.shape[1]):wk = self.coef_[k]self.coef_[k] = 0p_k = X[:, k] @ (y - X @ self.coef_)if p_k < -self.alpha / 2: w_k = (p_k + self.alpha / 2) / z[k]elif p_k > self.alpha / 2: w_k = (p_k - self.alpha / 2) / z[k]else: w_k = 0tmp[k] = w_kself.coef_[k] = wk if np.linalg.norm(self.coef_ - tmp) < self.e:break self.coef_ = tmp return self
Ridge
class Ridge(LinearModel): ''' Ridge Regression. ''' def __init__(self, alpha=1.0): self.alpha = alpha super().__init__() def fit(self, X, y): ''' :param X_: shape = (n_samples + 1, n_features) :param y: shape = (n_samples]) :return: self ''' self.scaler.fit(X) X = self.scaler.transform(X) X = np.c_[np.ones(X.shape[0]), X] self.coef_ = np.linalg.inv( X.T @ X + self.alpha * np.eye(X.shape[1])) @ X.T @ y return self
測試代碼
import matplotlib.pyplot as pltimport numpy as npdef gen_reg_data(): X = np.arange(0, 45, 0.1) X = X + np.random.random(size=X.shape[0]) * 20 y = 2 * X + np.random.random(size=X.shape[0]) * 20 + 10 return X, ydef test_linear_regression(): clf = LinearRegression() X, y = gen_reg_data() clf.fit(X, y) plt.plot(X, y, ’.’) X_axis = np.arange(-5, 75, 0.1) plt.plot(X_axis, clf.predict(X_axis)) plt.title('Linear Regression') plt.show()def test_lasso(): clf = Lasso() X, y = gen_reg_data() clf.fit(X, y) plt.plot(X, y, ’.’) X_axis = np.arange(-5, 75, 0.1) plt.plot(X_axis, clf.predict(X_axis)) plt.title('Lasso') plt.show()def test_ridge(): clf = Ridge() X, y = gen_reg_data() clf.fit(X, y) plt.plot(X, y, ’.’) X_axis = np.arange(-5, 75, 0.1) plt.plot(X_axis, clf.predict(X_axis)) plt.title('Ridge') plt.show()
測試效果
更多機器學習代碼,請訪問 https://github.com/WiseDoge/plume
以上就是Python 實現 3 種回歸模型(Linear Regression,Lasso,Ridge)的示例的詳細內容,更多關于Python 實現 回歸模型的資料請關注好吧啦網其它相關文章!
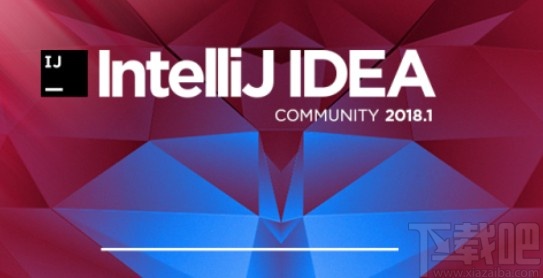