Python實現手勢識別
這是借鑒了github上的一個源程序,參考源:https://github.com/lzane/Fingers-Detection-using-OpenCV-and-Python
自己在這個基礎上做了一點修改補充后,可以實現手指指尖的檢測,并且可以在windows系統下通過判斷手指數目,來模擬鍵盤操作。下面直接上源程序,并做了詳細注釋,方便理解。
環境:python3.6+opencv3.4.0
代碼如下:
import cv2import numpy as npimport copyimport mathimport win32apiimport win32con# 參數cap_region_x_begin = 0.5 # 起點/總寬度cap_region_y_end = 0.8threshold = 60 # 二值化閾值blurValue = 41 # 高斯模糊參數bgSubThreshold = 50learningRate = 0# 變量isBgCaptured = 0 # 布爾類型, 背景是否被捕獲triggerSwitch = False # 如果正確,鍵盤模擬器將工作def printThreshold(thr): print('! Changed threshold to ' + str(thr))def removeBG(frame): #移除背景 fgmask = bgModel.apply(frame, learningRate=learningRate) #計算前景掩膜 kernel = np.ones((3, 3), np.uint8) fgmask = cv2.erode(fgmask, kernel, iterations=1) #使用特定的結構元素來侵蝕圖像。 res = cv2.bitwise_and(frame, frame, mask=fgmask) #使用掩膜移除靜態背景 return res# 相機/攝像頭camera = cv2.VideoCapture(0) #打開電腦自帶攝像頭,如果參數是1會打開外接攝像頭camera.set(10, 200) #設置視頻屬性cv2.namedWindow(’trackbar’) #設置窗口名字cv2.resizeWindow('trackbar', 640, 200) #重新設置窗口尺寸cv2.createTrackbar(’threshold’, ’trackbar’, threshold, 100, printThreshold)#createTrackbar是Opencv中的API,其可在顯示圖像的窗口中快速創建一個滑動控件,用于手動調節閾值,具有非常直觀的效果。while camera.isOpened(): ret, frame = camera.read() threshold = cv2.getTrackbarPos(’threshold’, ’trackbar’) #返回滑動條上的位置的值(即實時更新閾值) # frame = cv2.cvtColor(frame,cv2.COLOR_RGB2YCrCb) frame = cv2.bilateralFilter(frame, 5, 50, 100) # 雙邊濾波 frame = cv2.flip(frame, 1) # 翻轉 0:沿X軸翻轉(垂直翻轉) 大于0:沿Y軸翻轉(水平翻轉) 小于0:先沿X軸翻轉,再沿Y軸翻轉,等價于旋轉180° cv2.rectangle(frame, (int(cap_region_x_begin * frame.shape[1]), 0),(frame.shape[1], int(cap_region_y_end * frame.shape[0])), (0, 0, 255), 2) #畫矩形框 frame.shape[0]表示frame的高度 frame.shape[1]表示frame的寬度 注:opencv的像素是BGR順序 cv2.imshow(’original’, frame) #經過雙邊濾波后的初始化窗口 #主要操作 if isBgCaptured == 1: # isBgCaptured == 1 表示已經捕獲背景 img = removeBG(frame) #移除背景 img = img[0:int(cap_region_y_end * frame.shape[0]),int(cap_region_x_begin * frame.shape[1]):frame.shape[1]] # 剪切右上角矩形框區域 cv2.imshow(’mask’, img) gray = cv2.cvtColor(img, cv2.COLOR_BGR2GRAY) #將移除背景后的圖像轉換為灰度圖 blur = cv2.GaussianBlur(gray, (blurValue, blurValue), 0) #加高斯模糊 cv2.imshow(’blur’, blur) ret, thresh = cv2.threshold(blur, threshold, 255, cv2.THRESH_BINARY) #二值化處理 cv2.imshow(’binary’, thresh) # get the coutours thresh1 = copy.deepcopy(thresh) _, contours, hierarchy = cv2.findContours(thresh1, cv2.RETR_TREE, cv2.CHAIN_APPROX_SIMPLE) #尋找輪廓 注:這里的’_’用作變量名稱,_表示一個變量被指定了名稱,但不打算使用。 length = len(contours) maxArea = -1 if length > 0: for i in range(length): # 找到最大的輪廓(根據面積)temp = contours[i]area = cv2.contourArea(temp) #計算輪廓區域面積if area > maxArea: maxArea = area ci = i res = contours[ci] #得出最大的輪廓區域 hull = cv2.convexHull(res) #得出點集(組成輪廓的點)的凸包 drawing = np.zeros(img.shape, np.uint8) cv2.drawContours(drawing, [res], 0, (0, 255, 0), 2) #畫出最大區域輪廓 cv2.drawContours(drawing, [hull], 0, (0, 0, 255), 3) #畫出凸包輪廓 moments = cv2.moments(res) # 求最大區域輪廓的各階矩 center = (int(moments[’m10’] / moments[’m00’]), int(moments[’m01’] / moments[’m00’])) cv2.circle(drawing, center, 8, (0,0,255), -1) #畫出重心 fingerRes = [] #尋找指尖 max = 0; count = 0; notice = 0; cnt = 0 for i in range(len(res)):temp = res[i]dist = (temp[0][0] -center[0])*(temp[0][0] -center[0]) + (temp[0][1] -center[1])*(temp[0][1] -center[1]) #計算重心到輪廓邊緣的距離if dist > max: max = dist notice = iif dist != max: count = count + 1 if count > 40: count = 0 max = 0 flag = False #布爾值 if center[1] < res[notice][0][1]: #低于手心的點不算 continue for j in range(len(fingerRes)): #離得太近的不算 if abs(res[notice][0][0]-fingerRes[j][0]) < 20 :flag = Truebreak if flag : continue fingerRes.append(res[notice][0]) cv2.circle(drawing, tuple(res[notice][0]), 8 , (255, 0, 0), -1) #畫出指尖 cv2.line(drawing, center, tuple(res[notice][0]), (255, 0, 0), 2) cnt = cnt + 1 cv2.imshow(’output’, drawing) print(cnt) if triggerSwitch is True:if cnt >= 3: print(cnt) # app(’System Events’).keystroke(’ ’) # simulate pressing blank space win32api.keybd_event(32, 0, 0, 0) # 空格鍵位碼是32 win32api.keybd_event(32, 0, win32con.KEYEVENTF_KEYUP, 0) # 釋放空格鍵 # 輸入的鍵盤值 k = cv2.waitKey(10) if k == 27: # 按下ESC退出 break elif k == ord(’b’): # 按下’b’會捕獲背景 bgModel = cv2.createBackgroundSubtractorMOG2(0, bgSubThreshold) #Opencv集成了BackgroundSubtractorMOG2用于動態目標檢測,用到的是基于自適應混合高斯背景建模的背景減除法。 isBgCaptured = 1 print(’!!!Background Captured!!!’) elif k == ord(’r’): # 按下’r’會重置背景 bgModel = None triggerSwitch = False isBgCaptured = 0 print(’!!!Reset BackGround!!!’) elif k == ord(’n’): triggerSwitch = True print(’!!!Trigger On!!!’)
運行程序操作:運行程序后,按下鍵盤的 b 鍵就可以捕獲背景了
運行結果:
注:模擬點擊空格鍵部分并未展示出來,有興趣的可以嘗試一下(按下n鍵就可以模擬鍵盤操作了)
補:該程序受光線影響其實較大,只有在單調背景小效果很好。
-------------------補充----------------------
后期再運行該程序的時候發現有一個錯誤,如下:
原因:opencv版本的原因,在opencv 4.0.0版本后,findContours的返回值只有contours, hierarchy兩個參數,不再有三個參數了!
解決辦法:
方法一:
更換opencv的版本
方法二:
將代碼 _,contours, hierarchy = cv2.findContours(thresh1, cv2.RETR_TREE, cv2.CHAIN_APPROX_SIMPLE) 改為 contours, hierarchy = cv2.findContours(thresh1, cv2.RETR_TREE, cv2.CHAIN_APPROX_SIMPLE) 即可!
以上就是Python實現手勢識別的詳細內容,更多關于Python 手勢識別的資料請關注好吧啦網其它相關文章!
相關文章:
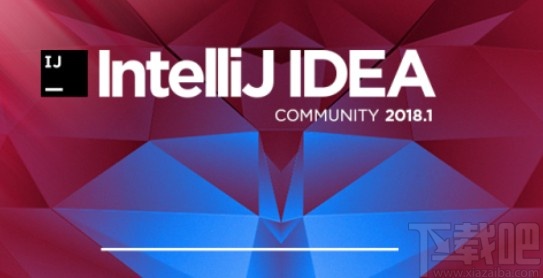